It was just five years ago that University of Washington atmospheric sciences professor Dale Durran published a paper with a title asking “Can Machines Learn to Predict Weather?”
At the time, many researchers were answering “no” to that question given the tremendous complexity of weather forecasting. But Durran and his co-authors saw promise in a fast-evolving technology and were already breaking ground in its application.
“The prospects for the development of much more accurate deep learning weather forecast models, based on much more information about the initial atmospheric state, appears excellent,” the Seattle-area scientists wrote.
Their prediction was spot on.
This summer, Durran and fellow researchers at Nvidia announced a tool called StormCast that can quickly and accurately predict thunderstorms and rainfall pinpointed to a range of just a couple of miles. StormCast, which relies on artificial intelligence and generative AI, can not only run faster than existing systems, it can also operate on much simpler machines that are more efficient at computing and use significantly less energy.
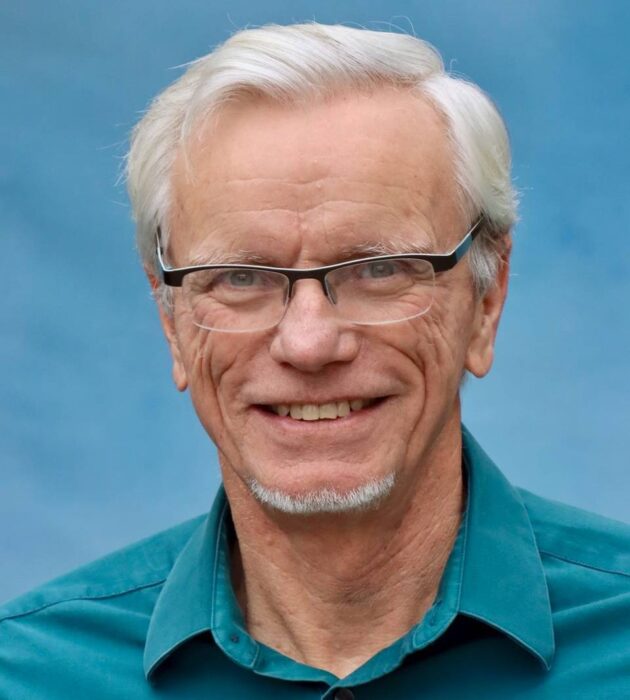
“It’s a very, very rapid and exciting change in how we can do forecasting,” Durran said in a GeekWire interview.
The tool is increasingly important as extreme weather events that include flooding, hailstorms and record-breaking temperatures become more common as the planet continues warming.
StormCast was created by a team largely from Nvidia, the longtime chip maker that is capitalizing on major tailwinds from the AI boom. Durran works primarily with the UW, but also has a research position with California-based Nvidia.
The group, which included researchers from Lawrence Berkeley National Laboratory and the University of Minnesota, in August released a preprint article on StormCast that has not undergone formal peer review.
The current U.S. benchmark for short-term weather predictions comes from the National Oceanic Atmospheric Administration (NOAA) and its High-Resolution Rapid Refresh (HRRR) modeling system. HRRR relies on numerical weather prediction for its forecasts, which requires a huge amount of computational power and it has limitations on the data inputs it can successfully incorporate.
Training the StormCast model took significant compute power and energy, but using it requires much less of both.
And while the NOAA model slowly cranks out a single forecast, StormCast works by rapidly running five different simulations that tweak the initial atmospheric conditions and parameters within the model using generative AI. The outcome is an ensemble of the simulations that can outperform a sole run on HRRR, according to StormCast researchers. StormCast is optimized for local forecasts covering current conditions to six hours out, with results up to 12 hours out.
“We’re able to do a better job over the first few hours to estimate where the rain is going to likely occur,” Durran said.
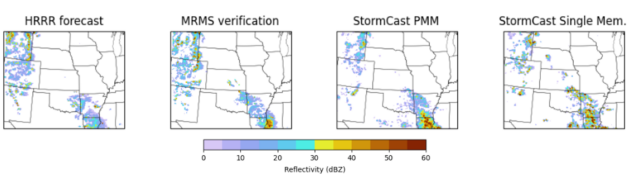
There are plans for StormCast to make better use of real-time radar and satellite information on clouds, for example, to improve its results.
NOAA is likewise working deliver more accurate forecasts. This week, NOAA and the U.S. Department of Commerce announced a $100 million investment in a new, high-performance computer system named Rhea that will bolster its use of AI in weather, climate and wildfire forecasting, plus other applications. The funding comes from the Bipartisan Infrastructure Law and Inflation Reduction Act.
AI is increasingly being incorporated into forecasting, with much of the focus on tools used for global predictions that stretch out 10 days. That includes work by the European Centre for Medium-Range Weather Forecasts and Microsoft’s Aurora, which provides forecasts on smoke and weather. Planette, a climate tech startup with Pacific Northwest roots, is using AI for forecasting weather and climate on the scale of next month to five years.
Durran doesn’t see StormCast as replacement for HRRR and other resources, but as a compliment to them or potentially a good starting point for forecasting.
The UW scientist credits Jonathan Weyn, one of his former graduate students and co-author on the 2019 publication, for helping alert him to AI’s potential in atmospheric science. The paper’s third author was Rich Caruana, a Microsoft Research scientist.
“We were definitely out in the lead,” said Durran. What’s going on now, he added, “it’s very exciting.”